苏ICP备112451047180号-6
可视化方法在民航旅客服务推荐中的应用研究
摘 要
目前,由于中国国内高铁的覆盖和高速的发展,以及航空公司之间的激烈竞争导致利润连续下滑,众多航空公司将推广附加服务以取得额外的收益成为取得差异化竞争优势的重要手段。本文从可视化角度首先对旅客出行行为特征不确定性进行可视分析,掌握旅客兴趣迁移;然后通过界面设计辅助用户交互地选择推荐的附加服务,提升推荐的有效性。主要工作包括以下方面:第一,民航旅客数据集的处理。对民航旅客订票数据集进行过滤、填充,确定信息属性,保证可视分析和推荐工作的顺利进行。
第二,民航旅客出行行为特征不确定性度量与可视分析。首先利用信息熵度量方法得到每个用户出行不确定性和属性不确定性的度量结果。针对民航旅客数据多维度的特点,采用平行坐标图对原数据进行表达,得到了数据在各个属性下取值的分布情况。然后,为了解决数据较多时重叠的折线会影响用户对属性轴上取值多少的判断,本文引入直方图嵌套到平行坐标上统计属性值分布情况。其次,为了探索旅客群体的特征,使用了适用于多变量对比分析的雷达图。最后,使用适用于多元数据可视化的像素图方法对出行地点进行了显示分析。通过对数据的处理和可视化,可以直观地获得旅客群体和个体在不同属性上的偏好情况,为后续附加服务推荐中提供个性化的服务打下了基础。
第三,民航旅客出行附加服务的推荐。利用基于用户的协同过滤推荐算法得到推荐结果,使用矩形树图的可视化方法对推荐结果进行展示,在推荐种类和推荐程度上都有较好地表现。并且利用节点-链接图对推荐结果进行解释,辅以缩放和选择的交互功能,支持用户查看近邻以及历史购买记录的相关情况,从而做出推荐选择。
最后,描述了可视分析与推荐实验系统的实现。
关键词:民航旅客出行数据;不确定性度量;可视分析;民航附加服务推荐
Abstract
It’s an strategic trends for airlines to provide additional services for extra yield under the background of increasing competition and gradually decreasing profit, which will obtain the differentiated competitive advantage. On the one hand, the uncertainty of passengers’ travel behavior have been analyzed by visual methods for mastering the condition of passengers’ interest migration. On the other hand, users can select recommended additional services interactively through the interface design, which can improve the effectiveness of recommendations. The main work includes the following aspects:
Firstly, the processing of the civil aviation passenger data set. In order to make sure the analysis process smoothly, the existing bookings data set of civil aviation passenger should be filtered and filled to ensure the visual analysis. Then the information attributes involved in the processing of visual analysis and recommendation should also be determined, and data object model is built for the convenience of the follow-up work.
Secondly, uncertainty measurement and visual analysis of the civil aviation passenger travel characteristics. First of all, uncertainty results of each passenger and attribute is measured on the theory of entropy. Then, according to the multidimensional characteristics of the civil aviation passenger data, parallel coordinates is used to express the original data to obtain the data’s distribution under each attribute axis. In order to solve the overlap lines under big scale data which affect the user’s judgment on attribute values in the axes, the histogram nested in the parallel coordinates is introduced. And then, to explore the characteristics of passenger groups, the radar map is applied for multivariate analysis. Finally pixel figure is applied to explore the travel locations which is an effective method for multivariate data. The passenger groups and individual preferences on different attributes can be directly observed through the data processing and visualization, which lays the foundation for subsequent additional services provided by the recommender system.
Thirdly, additional services recommendation of the civil aviation passenger travel. The recommendations are displayed in a tree-map based on the collaborative filtering algorithm, which has a better performance in the recommended types and recommended degree. And node - link diagram is applied to explain the result of the recommendation with scaling and choose function, which allows users to view the neighbors and history purchase records of relevant information, so as to make recommendations.
Finally, the visual analysis and recommender experiment system is described.
Keywords: Air Passenger Travel Data; Uncertainty Measurement; Visual Analysis; auxiliary service of civil aviation
目 录
摘 要 I
Abstract II
目 录 IV
第一章 绪论 1
1.1 研究背景与意义 1
1.2 国内外研究动态 2
1.2.1 不确定性度量理论 2
1.2.2 可视化方法在推荐系统的应用研究 3
1.3 本文的研究内容与组织结构 6
第二章 基础知识 8
2.1 基于信息熵的数据不确定性方法 8
2.1.1 信息系统概念 8
2.1.2 信息熵的相关定义 8
2.2 几种常见的可视化方法 9
2.2.1 多维数据可视化 9
2.2.2 网络数据可视化 10
2.2.3 层次数据可视化 11
2.2.4 交互方法介绍 11
2.3 本章小结 12
第三章 民航旅客数据处理 13
3.1 民航旅客订票数据集简介 13
3.2 民航旅客订票数据处理 13
3.2.1 数据过滤 13
3.2.2 数据填充 15
3.2.3 属性选取 16
3.2.4 附加服务评分数据集转化 16
3.3 本章小结 17
第四章 基于不确定性度量的民航旅客数据特征可视分析 18
4.1 不确定性度量 18
4.2 基于改进的平行坐标图表示的分析 19
4.2.1 普通平行坐标图表示 19
4.2.2 改进平行坐标图表示 21
4.3 基于雷达图表示的分析 22
4.4 基于像素图表示的分析 24
4.5 交互方法应用 25
4.6 本章小结 27
第五章 基于可视分析的民航旅客服务推荐 28
5.1 附加服务推荐可视化 28
5.1.1 基于用户的协同过滤算法 28
5.1.2推荐结果可视化模型 30
5.1.3基于协同过滤方法推荐结果交互可视化模型 31
5.2在民航旅客附加服务数据中的应用 32
5.2.1 推荐结果展示 32
5.2.2 推荐过程的可视化 33
5.2.3 用户交互 37
5.2.4 推荐结果分析 39
5.3本章小结 40
第六章 实验平台的设计与实现 41
6.1 实验平台设计框架 41
6.2 相关功能模块说明 42
6.2.1 文件读取模块 42
6.2.2 不确定性可视化方法选择模块 42
6.2.3 附加服务可视化推荐模块 43
6.2.4 人机交互设计模块 44
6.3 存在的不足 44
6.4 本章小结 45
第七章 工作总结与展望 46
7.1结论 46
7.2展望 46
参考文献 49
致谢 52
作者简介 53
[2] 陈为.数据可视化[M].北京:电子工业出版社,2013,12
[3] 周迪民, 林依勤. GIS属性数据不确定性及其传播研究[J]. 计算机工程, 2010, 36(6):250-252
[4] 周傲英,金澈清,王国仁等.不确定性数据管理技术研究综述[J],计算机学报, 2009,32(1):1-16
[5] 梅晓锋. 不确定性信息的度量及其应用[D]. 西北大学,2006
[6] 陈金广, 张芬, 马丽丽. 一种证据不确定性度量方法及其应用[J]. 计算机工程与应用, 2015(23)
[7] 魏巍, 魏琪, 王锋. 粗糙集的不确定性度量比较研究[J]. 南京大学学报:自然科学, 2015(4).
[8] Yager R R. Uncertainty representation using fuzzy measures[J]. IEEE Transactions on Systems Man & Cybernetics Part B Cybernetics A Publication of the IEEE Systems Man & Cybernetics Society, 2002, 32(1):13-20.
[9] Wierman M J. Measuring uncertainty in rough set theory. International Journal of General Systems,1999, 28(4):283-297.
[10] 杨本植. 基于粗糙集的电子商务推荐及可视化研究与实现[D]. 北京邮电大学, 2010.
[11] SELASSIED,HELLER B,HEER J. Divided edge bundling for directional network data[J]. IEEE Trans Visualization and Computer Graphics, 2011.17(12):2354-2363.
[12] 李绪, 曹磊, 付磊. 社交网络数据个性化推荐的可视化方法[J]. 计算机工程, 2014, 40(3): 46-50.
[13] 刘芳. 信息可视化技术及应用研究[D]. 浙江大学,2013.
[14] Herlocker J L, Konstan J A, Riedl J. Explaining collaborative filtering recommendations[C]//Proceedings of the 2000 ACM conference on Computer supported cooperative work. ACM, 2000: 241-250.
[15] Chen L, Tsoi H K. Users’ Decision Behavior in Recommender Interfaces: Impact of Layout Design[C]//RecSys’ 11 Workshop on Human Decision Making in Recommender Systems. 2011.
[16] Chen L, Pu P. Preference-based organization interfaces: aiding user critiques in recommender systems[M]//User Modeling 2007. Springer Berlin Heidelberg, 2007: 77-86.
[17] 魏丽芹.基于历史信息的就业推荐算法研究与可视化[D].山东大学,2013年
[18] 郝红红. 基于关联规则可视化技术的个性图书推荐系统研究[D].燕山大学,2012年
[19] O'Donovan J, Smyth B, Gretarsson B, et al. PeerChooser: visual interactive recommendation[C]//Proceedings of the SIGCHI Conference on Human Factors in Computing Systems. ACM, 2008: 1085-1088.
[20] Gretarsson B, O'Donovan J, Bostandjiev S, et al. Smallworlds: Visualizing social recommendations[C]//Computer Graphics Forum. Blackwell Publishing Ltd, 2010, 29(3): 833-842.
[21] Bostandjiev S, O'Donovan J, Höllerer T. Tasteweights: a visual interactive hybrid recommender system[C]//Proceedings of the sixth ACM conference on Recommender systems. ACM, 2012: 35-42
[22] Verbert K, Parra D, Brusilovsky P, et al. Visualizing recommendations to support exploration, transparency and controllability[C]//Proceedings of the 2013 international conference on Intelligent user interfaces. ACM, 2013: 351-362.
[23] 滕书华, 鲁敏, 张军,等. 信息系统中的熵理论和信息粒度[J]. 计算机工程与科学, 2012, 34(4):94-101.
[24] 解滨. 信息系统中的知识获取与不确定性度量的若干问题研究[D]. 河北师范大学, 2011
[25] Claessen J H T, Van Wijk J J. Flexible linked axes for multivariate data visualization[J]. Visualization and Computer Graphics, IEEE Transactions on, 2011, 17(12): 2310-2316.
[26] 陈谊, 李潇潇, 蔡进峰, 等. 基于类区间的多维数据可视化方法[J]. 系统仿真学报, 2013, 25(010): 2418-2423.
[27] 徐立婷.多维数据可视化[D]. 哈尔滨工程大学. 2010年
[28] 姚中华, 张龙飞, 宋汉辰. ParallelCluster: 一种时序多维数据的可视化方法[J]. 系统仿真学报, 2013, 25(009): 2135-2139.
[29] 雷琴琴. 面向像素的可视化技术研究[D]. 北京交通大学, 2008.
[30] 孙扬, 蒋远翔, 赵翔,等. 网络可视化研究综述[J]. 计算机科学, 2010, 37(2):12-18.
[31] Robertson G G, Mackinlay J D, Card S K. Cone Trees: animated 3D visualizations of hierarchical information[C]// Chi91 Conference on Human Factors in Computing Systems. ACM, 1991:189-194.
[32] 张昕, 袁晓如. 树图可视化[J]. 计算机辅助设计与图形学学报, 2012, 24(9):1113-1124.
[33] Daniel A. Keim . Informat ion Visualizat ion and Visual Dat a Mining[ J ] . IE EE Transactions on Visualizat ion and Comput er Graphics,2002, 7( 1) : 100- 107
[34] 邓银燕. 缺失数据的填充方法研究及实证分析[D]. 西北大学, 2010.
[35] 王宝丽. 信息系统中的不确定性度量与知识获取研究[D]. 山西大学, 2007.
[36] 陈谊, 蔡进峰, 石耀斌, 等. 基于平行坐标的多视图协同可视分析方法[J]. 系统仿真学报, 2013, 25(1): 81-86.
[37] 丁杰敏, 李伟波. 基于雷达图的多维数据可视化表示及特征分析[J]. 现代电子技术, 2010, 33(23):24-26.
[38] 李勇. 基于像素的可视化技术及其度量模型的研究[D]. 北京交通大学, 2010.
[39] Chen L, Pu P. Eye-Tracking study of user behavior in recommender interfaces[M]//User Modeling, Adaptation, and Personalization. Springer Berlin Heidelberg, 2010: 375-380.
[40] 刘迪.网页中交互式信息可视化设计研究[D].江南大学,2013年
[41] J Schaffer,T Höllerer,J O’Donovan.Hypothetical Recommendation: A Study of Interactive Profile Manipulation Behavior for Recommender Systems.
[42] 协同顾虑
[43] 任永功. 面向聚类的数据可视化方法及相关技术研究[D].东北大学.2006年
[44] 孙国道, 梁荣华, 何贤国,等. 高维时空房地产数据的可视分析[J]. 计算机辅助设计与图形学学报, 2013, 25(8):1169-1176.
[45] 孙国道, 梁荣华, 何贤国,等. 高维时空房地产数据的可视分析[J]. 计算机辅助设计与图形学学报, 2013, 25(8):1169-1176.
[46] 李代超, 吴升. 面向不同主题的犯罪大数据可视分析[J]. 地球信息科学学报, 2014(05):735-745.
[47] 雷辉, 陈海东, 徐佳逸, 等. 不确定性可视化综述[J]. 计算机辅助设计与图形学学报, 2013, 25(3): 294-303.
[48] Chen L, Wang F. Sentiment-enhanced explanation of product recommendations[C]//Proceedings of the companion publication of the 23rd international conference on World wide web companion. International World Wide Web Conferences Steering Committee, 2014: 239-240.
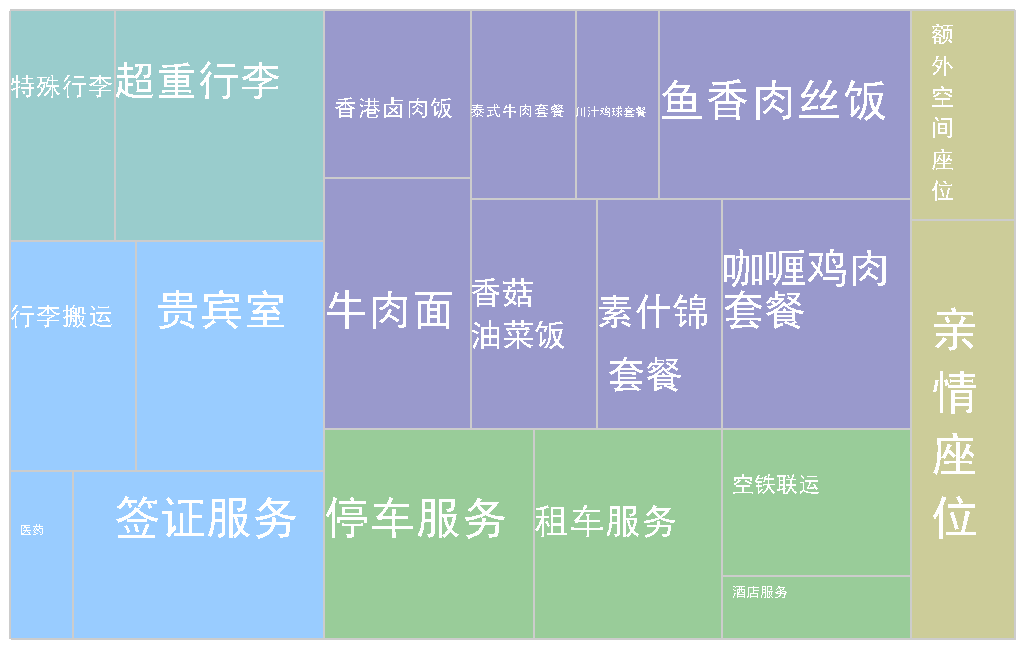
参考文献
[1] Dietmar Jannach. 推荐系统[M].北京:人民邮电出版社,2013-7[2] 陈为.数据可视化[M].北京:电子工业出版社,2013,12
[3] 周迪民, 林依勤. GIS属性数据不确定性及其传播研究[J]. 计算机工程, 2010, 36(6):250-252
[4] 周傲英,金澈清,王国仁等.不确定性数据管理技术研究综述[J],计算机学报, 2009,32(1):1-16
[5] 梅晓锋. 不确定性信息的度量及其应用[D]. 西北大学,2006
[6] 陈金广, 张芬, 马丽丽. 一种证据不确定性度量方法及其应用[J]. 计算机工程与应用, 2015(23)
[7] 魏巍, 魏琪, 王锋. 粗糙集的不确定性度量比较研究[J]. 南京大学学报:自然科学, 2015(4).
[8] Yager R R. Uncertainty representation using fuzzy measures[J]. IEEE Transactions on Systems Man & Cybernetics Part B Cybernetics A Publication of the IEEE Systems Man & Cybernetics Society, 2002, 32(1):13-20.
[9] Wierman M J. Measuring uncertainty in rough set theory. International Journal of General Systems,1999, 28(4):283-297.
[10] 杨本植. 基于粗糙集的电子商务推荐及可视化研究与实现[D]. 北京邮电大学, 2010.
[11] SELASSIED,HELLER B,HEER J. Divided edge bundling for directional network data[J]. IEEE Trans Visualization and Computer Graphics, 2011.17(12):2354-2363.
[12] 李绪, 曹磊, 付磊. 社交网络数据个性化推荐的可视化方法[J]. 计算机工程, 2014, 40(3): 46-50.
[13] 刘芳. 信息可视化技术及应用研究[D]. 浙江大学,2013.
[14] Herlocker J L, Konstan J A, Riedl J. Explaining collaborative filtering recommendations[C]//Proceedings of the 2000 ACM conference on Computer supported cooperative work. ACM, 2000: 241-250.
[15] Chen L, Tsoi H K. Users’ Decision Behavior in Recommender Interfaces: Impact of Layout Design[C]//RecSys’ 11 Workshop on Human Decision Making in Recommender Systems. 2011.
[16] Chen L, Pu P. Preference-based organization interfaces: aiding user critiques in recommender systems[M]//User Modeling 2007. Springer Berlin Heidelberg, 2007: 77-86.
[17] 魏丽芹.基于历史信息的就业推荐算法研究与可视化[D].山东大学,2013年
[18] 郝红红. 基于关联规则可视化技术的个性图书推荐系统研究[D].燕山大学,2012年
[19] O'Donovan J, Smyth B, Gretarsson B, et al. PeerChooser: visual interactive recommendation[C]//Proceedings of the SIGCHI Conference on Human Factors in Computing Systems. ACM, 2008: 1085-1088.
[20] Gretarsson B, O'Donovan J, Bostandjiev S, et al. Smallworlds: Visualizing social recommendations[C]//Computer Graphics Forum. Blackwell Publishing Ltd, 2010, 29(3): 833-842.
[21] Bostandjiev S, O'Donovan J, Höllerer T. Tasteweights: a visual interactive hybrid recommender system[C]//Proceedings of the sixth ACM conference on Recommender systems. ACM, 2012: 35-42
[22] Verbert K, Parra D, Brusilovsky P, et al. Visualizing recommendations to support exploration, transparency and controllability[C]//Proceedings of the 2013 international conference on Intelligent user interfaces. ACM, 2013: 351-362.
[23] 滕书华, 鲁敏, 张军,等. 信息系统中的熵理论和信息粒度[J]. 计算机工程与科学, 2012, 34(4):94-101.
[24] 解滨. 信息系统中的知识获取与不确定性度量的若干问题研究[D]. 河北师范大学, 2011
[25] Claessen J H T, Van Wijk J J. Flexible linked axes for multivariate data visualization[J]. Visualization and Computer Graphics, IEEE Transactions on, 2011, 17(12): 2310-2316.
[26] 陈谊, 李潇潇, 蔡进峰, 等. 基于类区间的多维数据可视化方法[J]. 系统仿真学报, 2013, 25(010): 2418-2423.
[27] 徐立婷.多维数据可视化[D]. 哈尔滨工程大学. 2010年
[28] 姚中华, 张龙飞, 宋汉辰. ParallelCluster: 一种时序多维数据的可视化方法[J]. 系统仿真学报, 2013, 25(009): 2135-2139.
[29] 雷琴琴. 面向像素的可视化技术研究[D]. 北京交通大学, 2008.
[30] 孙扬, 蒋远翔, 赵翔,等. 网络可视化研究综述[J]. 计算机科学, 2010, 37(2):12-18.
[31] Robertson G G, Mackinlay J D, Card S K. Cone Trees: animated 3D visualizations of hierarchical information[C]// Chi91 Conference on Human Factors in Computing Systems. ACM, 1991:189-194.
[32] 张昕, 袁晓如. 树图可视化[J]. 计算机辅助设计与图形学学报, 2012, 24(9):1113-1124.
[33] Daniel A. Keim . Informat ion Visualizat ion and Visual Dat a Mining[ J ] . IE EE Transactions on Visualizat ion and Comput er Graphics,2002, 7( 1) : 100- 107
[34] 邓银燕. 缺失数据的填充方法研究及实证分析[D]. 西北大学, 2010.
[35] 王宝丽. 信息系统中的不确定性度量与知识获取研究[D]. 山西大学, 2007.
[36] 陈谊, 蔡进峰, 石耀斌, 等. 基于平行坐标的多视图协同可视分析方法[J]. 系统仿真学报, 2013, 25(1): 81-86.
[37] 丁杰敏, 李伟波. 基于雷达图的多维数据可视化表示及特征分析[J]. 现代电子技术, 2010, 33(23):24-26.
[38] 李勇. 基于像素的可视化技术及其度量模型的研究[D]. 北京交通大学, 2010.
[39] Chen L, Pu P. Eye-Tracking study of user behavior in recommender interfaces[M]//User Modeling, Adaptation, and Personalization. Springer Berlin Heidelberg, 2010: 375-380.
[40] 刘迪.网页中交互式信息可视化设计研究[D].江南大学,2013年
[41] J Schaffer,T Höllerer,J O’Donovan.Hypothetical Recommendation: A Study of Interactive Profile Manipulation Behavior for Recommender Systems.
[42] 协同顾虑
[43] 任永功. 面向聚类的数据可视化方法及相关技术研究[D].东北大学.2006年
[44] 孙国道, 梁荣华, 何贤国,等. 高维时空房地产数据的可视分析[J]. 计算机辅助设计与图形学学报, 2013, 25(8):1169-1176.
[45] 孙国道, 梁荣华, 何贤国,等. 高维时空房地产数据的可视分析[J]. 计算机辅助设计与图形学学报, 2013, 25(8):1169-1176.
[46] 李代超, 吴升. 面向不同主题的犯罪大数据可视分析[J]. 地球信息科学学报, 2014(05):735-745.
[47] 雷辉, 陈海东, 徐佳逸, 等. 不确定性可视化综述[J]. 计算机辅助设计与图形学学报, 2013, 25(3): 294-303.
[48] Chen L, Wang F. Sentiment-enhanced explanation of product recommendations[C]//Proceedings of the companion publication of the 23rd international conference on World wide web companion. International World Wide Web Conferences Steering Committee, 2014: 239-240.
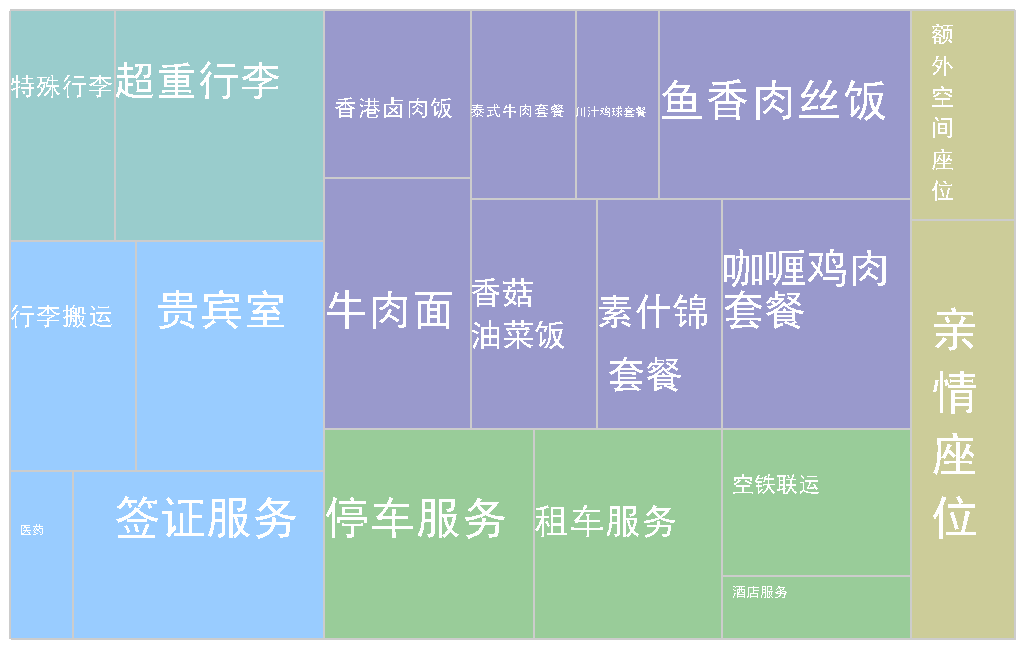