苏ICP备112451047180号-6
基于变分模态分解的柴油机噪声声源识别以及独立分量的鲁棒性分析
摘要
通过分离并识别柴油机的噪声声源,所得到的每一种柴油机具体部位发出的噪声可以作为柴油机降噪,工况监控以及故障诊断的依据。在柴油机噪声识别过程中发现,燃烧噪声和活塞撞击声在时间域和频率域上是相互重叠的。所以分离各种噪声的工作时极其复杂的。因此,这次试验中需要用到基于变分模态分解(VMD)的柴油机的噪声源定位方法、独立分量的鲁棒性分析(RobustICA)以及连续微波变换(CWT)。整个测试过程在半消声室中进行,测试对象是一台六缸柴油机。使用铅包线方法包裹1-5号气缸,只有6号气缸部分裸露,使得测试的噪声完全来自于六号缸而其余五个缸的噪声不会对测试产生干扰。首先对气缸顶部进行单通道噪声测量。然后利用变分模态分解算法将这一单通道噪声信号分解成多个变分模态组分。利用RobustICA算法对每个组分进行提纯,剔除无关的干扰信号。最后,运用连续微波变换并结合所学知识对分离后的结果进行进一步的识别。结果显示,通过运用上述系列方法来分离柴油机气缸顶部的噪声并对其进行识别,可以很好地分别得到单独的燃烧噪声和活塞撞击噪声。与EEMDRobustICA-CWT 法相比,通过上述方法可以得到更加精准、纯净的独立噪声分量,以及较少的干扰信号。
摘要
通过分离并识别柴油机的噪声声源,所得到的每一种柴油机具体部位发出的噪声可以作为柴油机降噪,工况监控以及故障诊断的依据。在柴油机噪声识别过程中发现,燃烧噪声和活塞撞击声在时间域和频率域上是相互重叠的。所以分离各种噪声的工作时极其复杂的。因此,这次试验中需要用到基于变分模态分解(VMD)的柴油机的噪声源定位方法、独立分量的鲁棒性分析(RobustICA)以及连续微波变换(CWT)。整个测试过程在半消声室中进行,测试对象是一台六缸柴油机。使用铅包线方法包裹1-5号气缸,只有6号气缸部分裸露,使得测试的噪声完全来自于六号缸而其余五个缸的噪声不会对测试产生干扰。首先对气缸顶部进行单通道噪声测量。然后利用变分模态分解算法将这一单通道噪声信号分解成多个变分模态组分。利用RobustICA算法对每个组分进行提纯,剔除无关的干扰信号。最后,运用连续微波变换并结合所学知识对分离后的结果进行进一步的识别。结果显示,通过运用上述系列方法来分离柴油机气缸顶部的噪声并对其进行识别,可以很好地分别得到单独的燃烧噪声和活塞撞击噪声。与EEMDRobustICA-CWT 法相比,通过上述方法可以得到更加精准、纯净的独立噪声分量,以及较少的干扰信号。
目录
摘要 2
1. 简介 2
2.基本理论 3
2.1.变分模态分解 3
2.2.独立分量的鲁棒性分析 4
2.3.连续微波变换 5
3.信号模拟 6
3.1.利用EMD、EEMD及VMD进行信号分离 6
3.2.运用FastICA和RobustICA进行信号分离 7
4.柴油机噪声试验 8
4.1.测试平台 8
4.2.试验前预处理 8
4.3.测试系统 9
5.柴油机噪声源分离和识别 10
5.1.VMD-RobustICA-CWT法 10
5.2.EEMD-RobustICA-CWT法 12
6.结论 13
1. 简介
柴油机是当今水陆交通工具的主要动力装置。其中柴油机噪声的控制也是如今降低噪声污染的主要研究方向。柴油机主要的噪声来源于燃烧噪声、机械噪声以及气体流动产生的噪声。如果在实际测量过程中测量的到的各部分混合噪声可以被分解,那么所得到的各噪声分量就可以为柴油机在降噪设计方面提供准确的信息,从而降低柴油机震动,减少噪声排放。
此外,柴油发动机的噪声包含着柴油机工作状态的重要信息,每个独立噪声源可以作为状态监测和故障诊断的基础(4、5)。近年来,许多研究人员采用多通道方法分离和识别柴油机的噪声源。比如,Badaoui et al .[6、7]利用循环维纳滤波和盲源分离算法来分离柴油机的燃烧噪声和活塞撞击噪声。
Xi et al.[8] 应用近场声强法和近场声全息术技术确定柴油机链式驱动系统的噪声和振动。Gu et al. [9] 利用声强、声学阵列法识别怠速状态下的发动机噪声。 Leclère et al. [10] 在怠速柴油机振幅调制现象的研究中引入了多通道频谱分析法。Lin et al. [11] 测量得到了发动机的多通道噪声信号。然后改进了盲源分离法使其可以适用于识别发动机的机械噪声和燃烧噪声。Blacodon and Lewy [12]在涡流式发动机的噪声识别中使用了三传感相干法。
当使用多通道方法确定柴油机的噪声来源,需要大量的传感器同时测量多个通道信号。传统的盲源分解法不能解决欠定的分离问题,同时传感器也是相当昂贵。为了在达到相同噪声源分离效果的前提下使用较少的传感器,测试人员在分离和定位噪声源时研究使用了单通道测量法。比如,Du et al. [13]在分离柴油机振动信号中使用了经验模态分解法(EMD)。但是在使用EMD法分析噪声信号过程中会产生边界效应和模态混叠的问题。
此外,Bi et al。[16]采用EEMD-RobustICA方法识别汽油机的噪声源。但EEMD(集成经验模态分解)方法仍出现模态混叠问题。另外,由于在每一次使用EMD法之前需要添加高斯白噪声,这个方法需要大量的时间。同时,确定所添加的高斯白噪声的幅值的工作也有很大的难度。由于柴油机中存在很多的噪声声源,分离出所有的噪声声源是不可能也是不必要的。目前,精准地分离出柴油机的燃烧噪声和活塞撞击声仍然是极其复杂的。
当活塞运动到上死点附近(TDC)、燃烧噪声和活塞敲击噪声在时间域和频率域相互重叠。因此,本文的目标是分离和识别柴油机的燃烧噪音和活塞敲击噪声。本次噪声实验在半消声室中进行,测试对象是WP10-240型柴油机。使用铅包线方法包裹1-5号气缸,只有6号气缸部分裸露。此外,为了排除空气流动产生的噪声,主要排放气体被连接到了实验室外的长管道中。最后,运用VMD-RobustICA-CWT来分离并识别六号缸的噪声源。
References
[1] Pietila G, Lim TC. Intelligent systems approaches to product sound quality evaluations – a review. Appl Acoust 2012;73(10):987–1002.
[2] Mao J, Hao ZY, Jing GX, et al. Sound quality improvement for a four-cylinder diesel engine by the block structure optimization. Appl Acoust 2013;74 (1):150–9.
[3] Liu H, Zhang J, Guo P, et al. Sound quality prediction for engine-radiated noise. Mech Syst Signal Process 2015;56:277–87.
[4] Li W, Parkin RM, Coy J, et al. Acoustic based condition monitoring of a diesel engine using self-organising map networks. Appl Acoust 2002;63(7):699–711.
[5] Jena DP, Panigrahi SN. Automatic gear and bearing fault localization using vibration and acoustic signals. Appl Acoust 2015;98:20–33.
[6] Badaoui ME, Danière J, Guillet F, et al. Separation of combustion noise and piston-slap in diesel engine—Part I: separation of combustion noise and piston-slap in diesel engine by cyclic wiener filtering. Mech Syst Signal Process 2005;19(6):1209–17.
[7] Servière C, Lacoume JL, Badaoui ME. Separation of combustion noise and piston-slap in diesel engine—Part II: separation of combustion noise and piston-slap using blind source separation methods. Mech Syst Signal Process 2005;19(6):1218–29.
[8] Xi J, Feng Z, Wang G, et al. Vibration and noise source identification methods for a diesel engine. J Mech Sci Technol 2015;29(1):181–9.
[9] Gu CS, Dong JH, Gao H. Apply two methods to measure engine noise source identification. Appl Mech Mater 2014;536–537:259–63.
[10] Leclère Q, Pézerat C, Laulagnet B, et al. Application of multi-channel spectral analysis to identify the source of a noise amplitude modulation in a diesel engine operating at idle. Appl Acoust 2005;66(7):779–98.
[11] Lin Y, Lin T. Engine noise of identification using an improved blind sources separation algorithm//2013. In: International conference on computing, networking and communications (ICNC). IEEE Computer Society; 2007. p. 759–63.
[12] Blacodon D, Lewy S. Source localization of turboshaft engine broadband noise using a three-sensor coherence method. J Sound Vib 2015;338:250–62.
[13] Du Xianfeng, Li Zhijun, Bi Fengrong, et al. Source separation of diesel engine vibration based on the empirical mode decomposition and independent component analysis. Chin J Mech Eng 2012;25(3):557–63.
[14] Yang Y, Cheng J, Zhang K. An ensemble local means decomposition method and its application to local rub-impact fault diagnosis of the rotor systems. Measurement 2012;45(3):561–70.
[15] Wu ZH, Huang NE. Ensemble empirical mode decomposition: a noise-assisted data analysis method. Adv Adaptive Data Anal 2011;1(01):1–41.
[16] Bi F, Li L, Zhang J, et al. Source identification of gasoline engine noise based on continuous wavelet transform and EEMD–RobustICA. Appl Acoust 2015;100:34–42.
[17] Dragomiretskiy K, Zosso D. Variational mode decomposition. IEEE Trans Signal Process 2014;62(3):531–44.
[18] Zarzoso V, Comon P. Robust independent component analysis by iterative maximization of the kurtosis contrast with algebraic optimal step size. IEEE Trans Neural Networks 2010;21(2):248–61.
[19] Flandrin P, Rilling G, Goncalves P. Empirical mode decomposition as a filter bank. IEEE Signal Process Lett 2004;11(2):112–4.
[20] Ur Rehman N, Mandic DP. Filter bank property of multivariate empirical mode decomposition. IEEE Trans Signal Process 2011;59(5):2421–6.
[21] Wu ZH, Huang NE. On the filtering properties of the empirical mode decomposition. Adv Adaptive Data Anal 2010;2(04):397–414.
[22] Hyvärinen A. Fast and robust fixed-point algorithms for independent component analysis. IEEE Trans Neural Networks 1999;10(3):626–34.
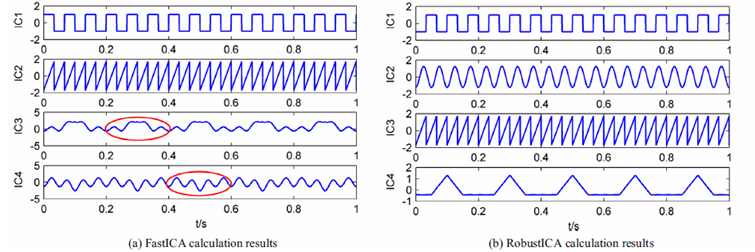
1. 简介
柴油机是当今水陆交通工具的主要动力装置。其中柴油机噪声的控制也是如今降低噪声污染的主要研究方向。柴油机主要的噪声来源于燃烧噪声、机械噪声以及气体流动产生的噪声。如果在实际测量过程中测量的到的各部分混合噪声可以被分解,那么所得到的各噪声分量就可以为柴油机在降噪设计方面提供准确的信息,从而降低柴油机震动,减少噪声排放。
此外,柴油发动机的噪声包含着柴油机工作状态的重要信息,每个独立噪声源可以作为状态监测和故障诊断的基础(4、5)。近年来,许多研究人员采用多通道方法分离和识别柴油机的噪声源。比如,Badaoui et al .[6、7]利用循环维纳滤波和盲源分离算法来分离柴油机的燃烧噪声和活塞撞击噪声。
Xi et al.[8] 应用近场声强法和近场声全息术技术确定柴油机链式驱动系统的噪声和振动。Gu et al. [9] 利用声强、声学阵列法识别怠速状态下的发动机噪声。 Leclère et al. [10] 在怠速柴油机振幅调制现象的研究中引入了多通道频谱分析法。Lin et al. [11] 测量得到了发动机的多通道噪声信号。然后改进了盲源分离法使其可以适用于识别发动机的机械噪声和燃烧噪声。Blacodon and Lewy [12]在涡流式发动机的噪声识别中使用了三传感相干法。
当使用多通道方法确定柴油机的噪声来源,需要大量的传感器同时测量多个通道信号。传统的盲源分解法不能解决欠定的分离问题,同时传感器也是相当昂贵。为了在达到相同噪声源分离效果的前提下使用较少的传感器,测试人员在分离和定位噪声源时研究使用了单通道测量法。比如,Du et al. [13]在分离柴油机振动信号中使用了经验模态分解法(EMD)。但是在使用EMD法分析噪声信号过程中会产生边界效应和模态混叠的问题。
此外,Bi et al。[16]采用EEMD-RobustICA方法识别汽油机的噪声源。但EEMD(集成经验模态分解)方法仍出现模态混叠问题。另外,由于在每一次使用EMD法之前需要添加高斯白噪声,这个方法需要大量的时间。同时,确定所添加的高斯白噪声的幅值的工作也有很大的难度。由于柴油机中存在很多的噪声声源,分离出所有的噪声声源是不可能也是不必要的。目前,精准地分离出柴油机的燃烧噪声和活塞撞击声仍然是极其复杂的。
当活塞运动到上死点附近(TDC)、燃烧噪声和活塞敲击噪声在时间域和频率域相互重叠。因此,本文的目标是分离和识别柴油机的燃烧噪音和活塞敲击噪声。本次噪声实验在半消声室中进行,测试对象是WP10-240型柴油机。使用铅包线方法包裹1-5号气缸,只有6号气缸部分裸露。此外,为了排除空气流动产生的噪声,主要排放气体被连接到了实验室外的长管道中。最后,运用VMD-RobustICA-CWT来分离并识别六号缸的噪声源。
References
[1] Pietila G, Lim TC. Intelligent systems approaches to product sound quality evaluations – a review. Appl Acoust 2012;73(10):987–1002.
[2] Mao J, Hao ZY, Jing GX, et al. Sound quality improvement for a four-cylinder diesel engine by the block structure optimization. Appl Acoust 2013;74 (1):150–9.
[3] Liu H, Zhang J, Guo P, et al. Sound quality prediction for engine-radiated noise. Mech Syst Signal Process 2015;56:277–87.
[4] Li W, Parkin RM, Coy J, et al. Acoustic based condition monitoring of a diesel engine using self-organising map networks. Appl Acoust 2002;63(7):699–711.
[5] Jena DP, Panigrahi SN. Automatic gear and bearing fault localization using vibration and acoustic signals. Appl Acoust 2015;98:20–33.
[6] Badaoui ME, Danière J, Guillet F, et al. Separation of combustion noise and piston-slap in diesel engine—Part I: separation of combustion noise and piston-slap in diesel engine by cyclic wiener filtering. Mech Syst Signal Process 2005;19(6):1209–17.
[7] Servière C, Lacoume JL, Badaoui ME. Separation of combustion noise and piston-slap in diesel engine—Part II: separation of combustion noise and piston-slap using blind source separation methods. Mech Syst Signal Process 2005;19(6):1218–29.
[8] Xi J, Feng Z, Wang G, et al. Vibration and noise source identification methods for a diesel engine. J Mech Sci Technol 2015;29(1):181–9.
[9] Gu CS, Dong JH, Gao H. Apply two methods to measure engine noise source identification. Appl Mech Mater 2014;536–537:259–63.
[10] Leclère Q, Pézerat C, Laulagnet B, et al. Application of multi-channel spectral analysis to identify the source of a noise amplitude modulation in a diesel engine operating at idle. Appl Acoust 2005;66(7):779–98.
[11] Lin Y, Lin T. Engine noise of identification using an improved blind sources separation algorithm//2013. In: International conference on computing, networking and communications (ICNC). IEEE Computer Society; 2007. p. 759–63.
[12] Blacodon D, Lewy S. Source localization of turboshaft engine broadband noise using a three-sensor coherence method. J Sound Vib 2015;338:250–62.
[13] Du Xianfeng, Li Zhijun, Bi Fengrong, et al. Source separation of diesel engine vibration based on the empirical mode decomposition and independent component analysis. Chin J Mech Eng 2012;25(3):557–63.
[14] Yang Y, Cheng J, Zhang K. An ensemble local means decomposition method and its application to local rub-impact fault diagnosis of the rotor systems. Measurement 2012;45(3):561–70.
[15] Wu ZH, Huang NE. Ensemble empirical mode decomposition: a noise-assisted data analysis method. Adv Adaptive Data Anal 2011;1(01):1–41.
[16] Bi F, Li L, Zhang J, et al. Source identification of gasoline engine noise based on continuous wavelet transform and EEMD–RobustICA. Appl Acoust 2015;100:34–42.
[17] Dragomiretskiy K, Zosso D. Variational mode decomposition. IEEE Trans Signal Process 2014;62(3):531–44.
[18] Zarzoso V, Comon P. Robust independent component analysis by iterative maximization of the kurtosis contrast with algebraic optimal step size. IEEE Trans Neural Networks 2010;21(2):248–61.
[19] Flandrin P, Rilling G, Goncalves P. Empirical mode decomposition as a filter bank. IEEE Signal Process Lett 2004;11(2):112–4.
[20] Ur Rehman N, Mandic DP. Filter bank property of multivariate empirical mode decomposition. IEEE Trans Signal Process 2011;59(5):2421–6.
[21] Wu ZH, Huang NE. On the filtering properties of the empirical mode decomposition. Adv Adaptive Data Anal 2010;2(04):397–414.
[22] Hyvärinen A. Fast and robust fixed-point algorithms for independent component analysis. IEEE Trans Neural Networks 1999;10(3):626–34.
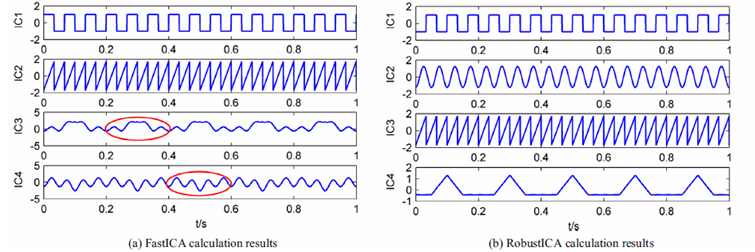