苏ICP备112451047180号-6
By Salil Prabhakar, Anil Jain
Fingerprint Matching:
Among all the biometric techniques, fingerprint-based identification is the oldest method which has been successfully used in numerous applications. Everyone is known to have unique, immutable fingerprints. A fingerprint is made of a series of ridges and furrows on the surface of the finger. The uniqueness of a fingerprint can be determined by the pattern of ridges and furrows as well as the minutiae points. Minutiae points are local ridge characteristics that occur at either a ridge bifurcation or a ridge ending. Fingerprint matching techniques can be placed into two categories: minutae-based and correlation based. Minutiae-based techniques first find minutiae points and then map their relative placement on the finger. However, there are some difficulties when using this approach. It is difficult to extract the minutiae points accurately when the fingerprint is of low quality. Also this method does not take into account the global pattern of ridges and furrows. The correlation-based method is able to overcome some of the difficulties of the minutiae-based approach. However, it has some of its own shortcomings. Correlation-based techniques require the precise location of a registration point and are affected by image translation and rotation.

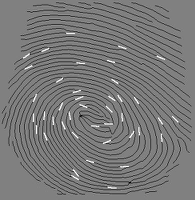
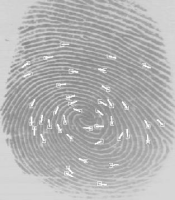
Fingerprint matching based on minutiae has problems in matching different sized (unregistered) minutiae patterns. Local ridge structures can not be completely characterized by minutiae. We are trying an alternate representation of fingerprints which will capture more local information and yield a fixed length code for the fingerprint. The matching will then hopefully become a relatively simple task of calculating the Euclidean distance will between the two codes
We are developing algorithms which are more robust to noise in fingerprint images and deliver increased accuracy in real-time. A commercial fingerprint-based authentication system requires a very low False Reject Rate (FAR) for a given False Accept Rate (FAR). This is very difficult to achieve with any one technique. We are investigating methods to pool evidence from various matching techniques to increase the overall accuracy of the system. In a real application, the sensor, the acquisition system and the variation in performance of the system over time is very critical. We are also field testing our system on a limited number of users to evaluate the system performance over a period of time.
Fingerprint Classification:
Large volumes of fingerprints are collected and stored everyday in a wide range of applications including forensics, access control, and driver license registration. An automatic recognition of people based on fingerprints requires that the input fingerprint be matched with a large number of fingerprints in a database (FBI database contains approximately 70 million fingerprints!). To reduce the search time and computational complexity, it is desirable to classify these fingerprints in an accurate and consistent manner so that the input fingerprint is required to be matched only with a subset of the fingerprints in the database.
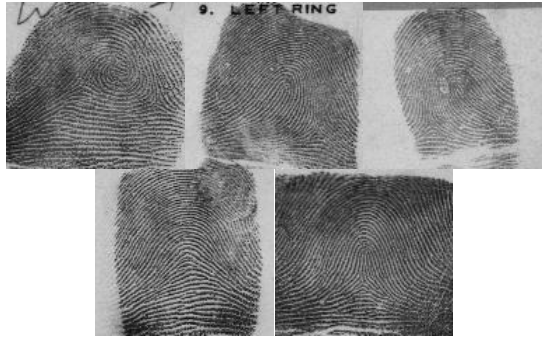
Fingerprint classification is a technique to assign a fingerprint into one of the several pre-specified types already established in the literature which can provide an indexing mechanism. Fingerprint classification can be viewed as a coarse level matching of the fingerprints. An input fingerprint is first matched at a coarse level to one of the pre-specified types and then, at a finer level, it is compared to the subset of the database containing that type of fingerprints only. We have developed an algorithm to classify fingerprints into five classes, namely, whorl, right loop, left loop, arch, and tented arch. The algorithm separates the number of ridges present in four directions (0 degree, 45 degree, 90 degree, and 135 degree) by filtering the central part of a fingerprint with a bank of Gabor filters. This information is quantized to generate a Finger Code which is used for classification. Our classification is based on a two-stage classifier which uses a K-nearest neighbor classifier in the first stage and a set of neural networks in the second stage. The classifier is tested on 4,000 images in the NIST-4 database. For the five-class problem, classification accuracy of 90% is achieved. For the four-class problem (arch and tented arch combined into one class), we are able to achieve a classification accuracy of 94.8%. By incorporating a reject option, the classification accuracy can be increased to 96% for the five-class classification and to 97.8% for the four-class classification when 30.8% of the images are rejected.
Fingerprint Image Enhancement
A critical step in automatic fingerprint matching is to automatically and reliably extract minutiae from the input fingerprint images. However, the performance of a minutiae extraction algorithm relies heavily on the quality of the input fingerprint images. In order to ensure that the performance of an automatic fingerprint identification verification system will be robust with respect to the quality of the fingerprint images, it is essential to incorporate a fingerprint enhancement algorithm in the minutiae extraction module. We have developed a fast fingerprint enhancement algorithm, which can adaptively improve the clarity of ridge and furrow structures of input fingerprint images based on the estimated local ridge orientation and frequency. We have evaluated the performance of the image enhancement algorithm using the goodness index of the extracted minutiae and the accuracy of an online fingerprint verification system. Experimental results show that incorporating the enhancement algorithms improves both the goodness index and the verification accuracy.
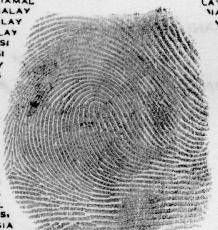
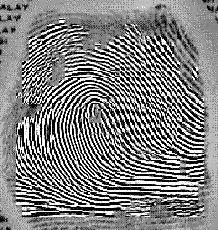
Fingerprint Identifies Arithmetic
The fingerprint technique of scans can divided into 2 types generally: identification system, such as AFIS(automatic fingerprint confirm system) and verification system, two kinds of system key of the differentiation is in the fingerprint template. verification the system equally needs to obtain fingerprint image, but this kind of technique doesn't keep complete fingerprint image and it just keeps through some particular datas that some calculate way processings chase a fingerprint in an opposite smaller template(250-1000 word byte).When these datas are pick up after, the fingerprint portrait won't be again keep and can't scan template to rebuild through a finger, either. For this, many companies with domestic and international in the last yearses and its think factory produced many arithmetic in digital ways .
Evaluating a excellently arithmetic which commercial and big area expand , not only need from the miscarriage of justice rate of normal regulations, refused to judge a rate, opposite accuracy, refused to ascend a rate etc. parameter to evaluate, a good cal arithmetic includes various, for example: Can enough filter in addition to fingerprint noise? Adapt different angle to press to press? Adapt different fingerprint quality? Whether in consideration of does the high speed match? Can filter a remnants remaining fingerprint information? Whether can order in the as far as possible little characteristic under identify? Adapt a fingerprint dissimilarity the variety of the season? Can handle a too dry or moist fingerprint? Can adapt a dissimilarity to press the pressure degree? Occupy a quite a little memory? Whether very low to the dependence of system? Whether can good movement under various different operation environments? Can conveniently transplant to go to single slice machine system? Can run out the quantity little characteristic to express a fingerprint information? Does the customer feel very comfortable? Whether the development system opened very much to expand a market? Whether through a great deal of test of fingerprint database? Can let the customer experience a transparent test? Have low-down business threshold? Whether have excellent of 1:N performance? Does the software connect whether matches international norm or not? Can adapt the portrait of different quality? Can provide to connect for customer good development? Etc.
The principle of fingerprint identifies arithmetic is after the image was picked up which is a high quality and has to be converted into an useful format for it. If image is ash degree, opposite more shallow part will be abandon, but opposite deeper part be become black. The pixel of ridge is been thin by 5-8 to arrive a pixel, so ability the precision position the ridge break point and diverged. Such as: A arithmetic possibility at inspectional image pick and get rid of a detail of the two close detail, because these two details neared too much, because of the scar formation, sweat liquid or dust cause of detail abnormality, the arithmetic is incapable for the dint to these circumstances. Perhaps, one fork to be located on an island form scar perpendicular cut through 2-3 ridges on(may be a false detail) perhaps a ridge.(may be scar formation or dust)All this possible details want to be abandon in this processing. Once a point is indeed settle down, its position be origin(0, 0) of X, Y axile, in the detail the point of the fixed position process, or the place ridge be square upward of the terminal point have a corner.(the circs when the arch break up point appeared will be more complicated)
指纹确认
Salil Prabhakar, Anil Jain
指纹匹配:
在所有的生物技术之中,指纹识别技术已经被成功地应用于很多场合。每个人都知道指纹具有唯一不可变的特性。一个人的指纹是由手指的表面上一系列脊和沟做成的。指纹的独特性是由脊和沟的式样和细节点决定,细节点是发生一个脊分叉或一个脊终止时的当地的特征点。
指纹匹配技术可以分为两种: 基于细节的和基于相互关系的。以细节为基础的技术首先发现细节点,然后在手指上映射出他们的相对位置。然而,当使用这种方法时还有一些困难,当指纹质量低的时候,正确地吸取细节点很困难。这一方法也不考虑全球的指纹的脊和沟的式样。以相互关系为基础的方法能够克服以细节为基础方法的一些困难。然而,它也有一些自己的缺点。以相互关系为基础的技术需要精确定位登记点而且被图像翻译和旋转所影响。
基于细节的指纹匹配在匹配不同尺寸(未注册的) 的细节式样的指纹方面有问题,当地的脊结构不能完全地拥有细节的特点。 我们正在尝试为指纹取得更多的当地数据并且产生一个固定的长度密码。这时匹配将会变成像希望的那样计算在这二个密码之间的欧几里得几何距离的相对简单工作。
我们正在发展更强健的在指纹图像中去除噪音性强,递送准确性强,实时性强的运算法则。一个商业的指纹确认系统需要非常低的错误率 (FAR) 一个可以接受的错误比率.(FAR)这对于任何的一项技术都是一个难点。我们正在研究各种不同的相配技术来增加系统的准确性。在一个实时的应用环境中或在传感器中,系统的执行时间变的越来越重要。我们也是把我们的系统实地试验在少数用户上一段时间来评估我们的系统。
指纹分类:
每天大量的指纹被收集存储应用在不同的场合,例如法医,通路控制和驾驶员执照登记。以指纹为基础的自动识别系统需要输入一个与数据库中相匹配的指纹 (联邦调查局数据库大约包含七千万个指纹!)。为了减少搜寻时间和计算的复杂性,需要对这些指纹按正确和一致的方式分类,以便输入指纹时只需要在数据库中找到其中匹配的一个子集而已。
指纹分类是一种可以预先分配一个指纹进入已经分类好的几个类型之中并提供一种分度装置的技术。指纹分类可以看作是大概的粗糙的指纹类型的相配。 一个输入指纹首先对预先存储的类型大致相配,然后,在详细的分析,把他和只包含那一个类型指纹的数据库的子集相比较。我们正在研究一种运算法则——把指纹分类为五个类型,即螺旋状纹、右回旋,左回旋,拱形,帐篷形。运算法则被一个Gabor过滤器分开过滤为四个方向(0 度、 45 度、 90 度和 135 度)。这些数据被量化产生作为分类的指纹编码。我们的分类以一个二阶段的分类器为基础。第一个阶段用一个K最近的分类器和第二个阶段用一组类神经网路分类器。在分类器NIST-4 数据库中测试了4,000个图像。 对于五种类型的问题,分类的准确性达到90%。对于四种类型的问题 (拱门和帐篷型组合在一个类型之内),我们能够达成94.8%的分类准确性。当合并不合格者选项时,分类准确性能达到为五种分类时增加到 96% 和至 97.8% ,为四种类型时 30.8% 的被拒绝。
指纹图像增强:
在自动指纹识别系统中一个重要的步骤是从输入的指纹图像中自动地而且可靠地吸取指纹细节。然而,这种获取指纹细节的计算法则很严重地依赖输入指纹图像的质量。为了确定自动指纹识别的效果,确认系统是对指纹质量的重要衡量系统,有必要将提高指纹运算法则的质量作为细节提取模块的一部分。我们已经设计出了一个快速识别指纹的运算法则, 能尽量地增强脊的清晰度,还能以输入指纹图像的沟结构为基础,估计当地的脊定方位和频率。我们已经使用被吸取的细节点的索引和在线指纹确认系统来评估图像运算法则的表现。实验的结果表示这种运算法则能提高和改善确认的准确性。
指纹识别技术的算法:
指纹扫描技术大体可分为两类:确认(identification)系统,如 AFIS(自动指纹确认系统)和核对(verification)系统,两种系统主要区别在指纹模板。核对 (verification) 系统同样需要获取指纹图象,但这种技术并不保存完整的指纹图象,它只是通过一些算法处理把指纹的一些特定的数据保存在一个相对较小的模板( 250-1000 字节)中。当这些数据被拾取后,指纹图象将不再被保存,也不能通过手指扫描模板来重建。为此,多年来国内外公司及其研究机构产生了许多数字化的算法。
评估一个优秀的能够商业化大面积推广的指纹算法,不仅要从常规的误判率,拒判率,相对准确率,拒登率等参数来评估,一个好的算法包括有许多方面,例如:能否够滤除指纹噪音?是否适应不同的角度去按压?是否适应不同的指纹质量?是否考虑到高速匹配?是否能够过滤残余指纹信息?是否能够在尽量少的特征点下识别?是否适应指纹不同季节的变化?是否能够处理过于干燥或湿润的指纹 ? 是否能够适应不同按压力度?是否占有非常少的内存?是否对系统的依赖性很低?是否能够在各种不同的操作环境下良好的运行 ? 是否可以方便的移植到单片机系统中去?是否可以用尽量少的特征表述指纹信息?客户是否感到很舒适?是否有非常开放的开发体系来推广市场?是否经过大量的指纹库的测试?是否能够让客户经历透明的测试 ? 是否具备非常低的商业门槛 ? 是否有优秀的 1:N 的表现?软件接口是否符合国际规范?是否能适应不同质量的图象?是否能够提供给用户良好的开发接口?等等
指纹识别技术算法的工作原理为当一个高质量的图象被拾取后,它必须被转换成一个有用的格式。如果图象是灰度图象,相对较浅的部分会被舍弃,而相对较深的部分被变成了黑色。脊的象素由 5 到 8 个被缩细到一个象素,这样就能精确定位脊断点和分岔了。如:一个算法可能在检索图象时剔除两个邻近细节中的一个细节,因为这两个细节太接近了,由于疤痕,汗液或灰尘导致的细节异常,算法对于这些情况是无能为力的。或者,一个分岔位于一个岛形痕之上(可能是错误细节)或者一个脊垂直穿过两到三个脊(可能是疤痕或灰尘)。所有这些可能的细节都要在这个处理过程中被舍弃。一旦一个点被确定下来,它的位置就是 X,Y 轴和原点(0 ,0),在细节点的定位过程中,与所在脊方向上的终点有一个夹角(拱形断点的情况法则将更复杂)。